New York City subway map after Sandy
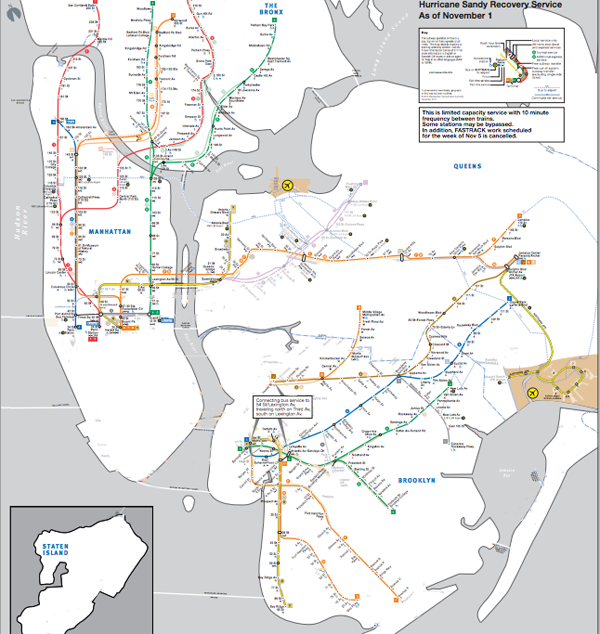
New York City ghosted lines subway map
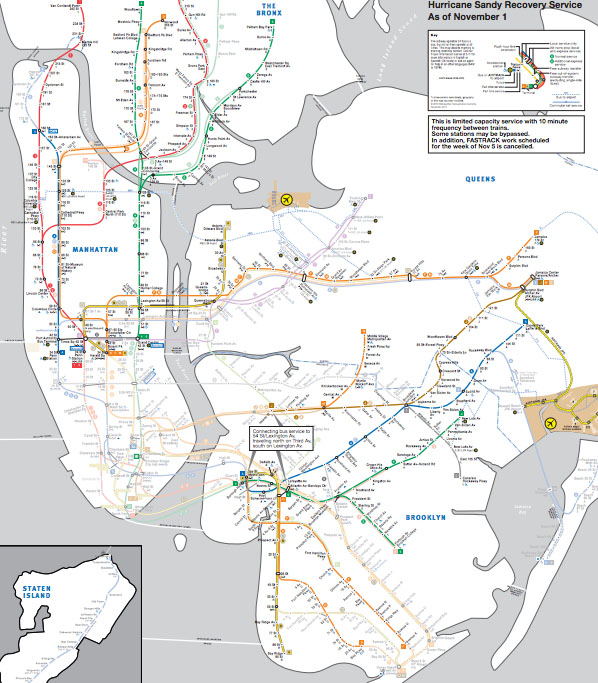
Not back to normal
For those of you living in New York, the subway map is probably familiar to you. For those who are not here, but are listening to reports, I thought I would post the maps to illustrate that the subways are not back to normal. The national broadcasts I listen to keep mentioning that the subways are coming back, which is true, but Sandy essentially knocked the center out of the network. What was once one network is now two networks with very strange structures. They connect, if at all, not through their abdomens like spiders’ legs, but at the very ends of their extremities and there is no recognizable abdomen.
The storm also knocked out some specific edges of the network, like the end of the A train that ran past JFK and into the Rockaways. Note to travelers: The New York City subway is no longer connected to JFK airport.
As of this morning, I am hearing different reports about the 7 train in Queens. It might be running to the connection with the F train according to WNYC, but the mta.info website does not yet reflect that change. I left the line partially ghosted in. There are no reports that the 7 train is running all the way into Manhattan.
Brooklyn
There is subway service between Queens and Manhattan but Brooklyn has been cut off almost completely.