In the past few months, I’ve posted about two works of long-form scholarship on the Quantified Self: Debora Lupton’s The Quantified Self and Gina Neff and Dawn Nufus’s Self-Tracking. Neff recently edited a volume of essays on QS (Quantified: Biosensing Technologies in Everyday Life, MIT 2016), but I’d like to take a not-so-brief break from reviewing books to address an issue that has been on my mind recently. Most texts that I read about the Quantified Self (be they traditional scholarship or more informal) refer to a meeting in 2007 at the house of Kevin Kelly for the official start to the QS movement. And while, yes, the name “Quantified Self” was coined by Kelly and his colleague Gary Wolf (the former founded Wired, the latter was an editor for the magazine), the practice of self-tracking obviously goes back much further than 10 years. Still, most historical references to the practice often point to Sanctorius of Padua, who, per an oft-cited study by consultant Melanie Swan, “studied energy expenditure in living systems by tracking his weight versus food intake and elimination for 30 years in the 16th century.” Neff and Nufus cite Benjamin Franklin’s practice of keeping a daily record of his time use. These anecdotal histories, however, don’t give us much in terms of understanding what a history of the Quantified Self is actually a history of. more...
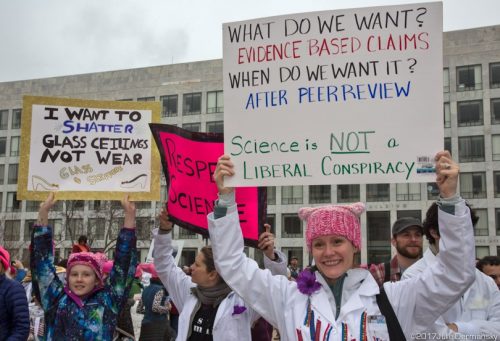
Science. Is. Political.
This concept will probably be easy to absorb for the regular readership at Cyborgology. It’s a topic that has been discussed here a time or two. Still, as truisms go, it is one of a very few that liberals and conservatives alike love to hate. The fantasy of apolitical science is a tempting one: an unbiased, socially distant capital-s Science that seeks nothing more than enlightenment, floating in a current events vacuum and unsullied by personal past experiences. It presupposes an objective reality, a universe of constants that can be catalogued, evaluated, and understood completely. But this view of science is a myth, one that has been thoroughly dissected in the social sciences. more...
Back in January, I wrote about Deborah Lupton’s The Quantified Self, a recent publication from Polity by the University of Canberra professor in Communication. In that post I mentioned that I planned to read another book on the QS movement from MIT Press, Self-Tracking by Gina Neff, a Communication scholar out of University of Washington, and Dawn Nafus, an anthropologist at Intel. And so I have. more...
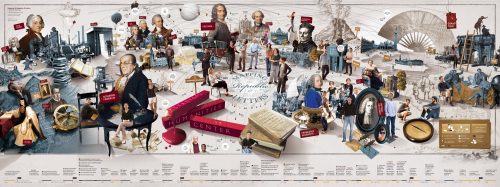
Over the past decade, theorizing about data and digital mediums has typically been kept to spaces like New Media Studies. The rise of Digital Humanities as a strictly empirical field cuts against this grain in a manner worth examining. Part I: The Hegemony of Data, discusses a longer history of information to evaluate the intuitive sense of objectivity that surrounds “Big Data”. Part II: The Heavens and Hells of the Web examines the initial beliefs in digital messianism as a method of eliminating moral and social problems, how they turned apocalyptic, and what lessons Digital Humanities should take from it. Part III: Digital Epistemology goes beyond critique and builds a sense in which anti-colonial, anti-capitalist, moral visions of a future may benefit and actually advance discourses through our experiences with digital tools and society.
more...

According to its author JG Ballard, Crash is ‘the first pornographic novel based on technology’. From bizarre to oddly pleasing, the book’s narrative drifts from one erotically charged description to another of mangled human bodies and machines fused together in the moment of a car crash. The “TV scientist”, Vaughan, and his motley crew of car crash fetishists seek out crashes in-the-making, even causing them, just for the thrill of it. The protagonist of the tale, a James Ballard, gets deeply entangled with Vaughan and his ragged band after his own experience of a crash. Vaughan’s ultimate sexual fantasy is to die in a head-on collision with the actress Elizabeth Taylor.
Like a STS scholar-version of Vaughan, I imagine what it may be like to arrive on the scene of a driverless car crash, and draw maps to understand what happened. Scenario planning is one kind of map-making to plan for ‘unthinkable futures’.
The ‘scenario’ is a phenomenon that became prominent during the Korean War, and through the following decades of the Cold War, to allow the US army to plan its strategy in the event of nuclear disaster. Peter Galison describes scenarios as a “literature of future war” “located somewhere between a story outline and ever more sophisticated role-playing war games”, “a staple of the new futurism”. Since then scenario-planning has been adopted by a range of organisations, and features in the modelling of risk and to identify errors. Galison cites the Boston Group as having written a scenario – their very first one- in which feminist epistemologists, historians, and philosophers of science running amok might present a threat to the release of radioactive waste from the Cold War (“A Feminist World, 2091”).
The applications of the Trolley Problem to driverless car crashes are a sort of scenario planning exercise. Now familiar to most readers of mainstream technology reporting, the Trolley problem is presented as a series of hypothetical situations with different outcomes derived from a pitting of consequentialism against deontological ethics. Trolley Problems are constructed as either/or scenarios where a single choice must be made. MIT’s Moral Machine project materialises this thought experiment with an online template in which the user has to complete scenarios in which she has to instruct the driverless car about which kinds of pedestrian to avoid in the case of brake failure: runaway criminals, pets, children, parents, athletes, old people, or fat people. Patrick Lin has a short animation describing the application of the Trolley Problem in the driverless car scenario.
The applications of the Trolley Problem, as well as the Pascalian Wager (Bhargava, 2016), are applications that attempt to create what-if scenarios. These scenarios guide the technical development of what has become both holy grail and a smokescreen in talking about autonomous driving (in the absence of an actual autonomous vehicle): how can we be sure that driverless car software will make the right assessment about the value of human lives in the case of an accident?
These scenarios and their outcomes is being referred to as the ‘ethics’ of autonomous driving. In the development of driverless cars we see an ambition for the development of what James Moor refers to as an ‘explicit ethical agent’ – one that is able to calculate the best action in an ethical dilemma. I resist this construction of ethics because I claim that relationships with complex machines, and the outcomes of our interactions with them, are not either/or, and are perhaps far more entangled, especially in crashes. There is an assumption in applications of Trolley Problems that crashes take place in certain specific ways, that ethical outcomes can be computed in a logical fashion, and that this will amount to an accountability for machine behaviour. There is an assumption that the driverless car is a kind of neoliberal subject itself, which can somehow account for its behaviour just as it might some day just drive itself (thanks to Martha Poon for this phrasing).
Crash scenarios are particular moments that are being planned for and against; what kinds of questions does the crash event allow us to ask about how we’re constructing relationships with and about machines with artificial intelligence technologies in them? I claim that when we say “ethics” in the context of hypothetical driverless car crashes, what we’re really saying “who is accountable for this?” or, “who will pay for this?”, or “how can we regulate machine intelligence?”, or “how should humans apply artificially intelligent technologies in different areas?”. Some of these questions can be drawn out in terms of an accident involving a Tesla semi-autonomous vehicle.
In May 2016, an ex-US Navy veteran was test-driving a Model S Tesla semi-autonomous vehicle. The test driver, who was allegedly watching a Harry Potter movie at the time with the car in ‘autopilot’ mode, drove into a large tractor trailer whose white surface was mistaken by the computer vision software for the bright sky. Thus it did not stop, and went straight into the truck. The fault, it seemed, was the driver’s for trusting the autopilot mode as the Tesla statement after the event says. Autopilot in the semi-autonomous car is perhaps misleading for those who go up in airplanes, so much so that the German government has told Tesla that it cannot use the word ‘autopilot’ in the German market.
In order to understand what might have happened in the Tesla case, it is necessary to look at applications of computer vision and machine learning in driverless cars. A driverless car is equipped with a variety of sensors and cameras that will record objects around it. These objects will be identified by specialised deep learning algorithms called neural nets. Neural nets are distinct in that they can be programmed to build internal models for identifying features of a dataset, and can learn how those features are related without being explicitly programmed to do so (NVIDIA 2016; Bojarski et al 2016).
Computer vision software makes an image of an object and breaks that image up into small parts – edges, lines, corners, colour gradients and so on. By looking at billions of images, neural nets can identify patterns in how combinations of edges, lines, corners and gradients come together to constitute different objects; this is its ‘model making’. The expectation is that such software can identify a ball, a cat, or a child, and that this identification will enable the car’s software to make a decision about how to react based on that identification.
Yet, this is a technology still in development and there is the possibility for much confusion. So, things that are yellow, or things that have faces and two ears on top of the head, which share features such as shape, or where edges, gradients, and lines come together, can be misread until the software sees enough examples that distinguish how things that are yellow, or things with two ears on the top of the head, are different. The more complex something is visually, without solid edges, curves or single colours; or if an object is fast, small, or flexible, the more difficult it is to read. So, computer vision in cars is shown to have a ‘bicycle problem‘ because bicycles are difficult to identify, do not always havea a specific, structured shape, and can move at different speeds.
In the case of the Tesla crash, the software misread the large expanse of the side of the trailer truck for the sky. It is possible that the machine learning was not well-trained enough to make the distinction. The Tesla crash suggests that there was both an error in the computer vision and machine learning software, as well as a lapse on the part of the test driver who misunderstood what autopilot meant. How, then, are these separate conditions to be understood as part of the dynamic that resulted in the crash? How might an ethics be imagined for this sort of crash that comes from an unfortunate entanglement of machine error and human error?
Looking away from driverless car crashes, and to aviation crashes instead, a specific narrative around the idea of accountability for crashes emerges. Galison, again, in his astounding chapter, An Accident of History, meticulously unpacks aviation crashes and how they are are investigated, documented and recorded. In doing so he makes the point that it is near impossible to escape the entanglements between human and machine actors involved in an accident. And that in attempting to identify how and why a crash occurred, we find a “recurrent strain to between a drive to ascribe final causation to human factors and an equally powerful, countervailing drive to assign agency to technological factors.” Galison finds that in accidents, human action and material agency are entwined to the point that causal chains both seem to terminate at particular, critical actions, as well as radiate out towards human interactions and organisational cultures. Yet, what is embedded in accident reporting is the desire for a “single point of culpability”, as Alexander Brown puts it, which never seems to come.
Brown’s own accounts of accidents and engineering at NASA, and Diane Vaughan’s landmark ethnography about the reasons for the Challenger Space Shuttle crash suggest the same: from organisational culture and bureaucratic processes, to faulty design, to a wide swathe of human errors, to the combinations of these, are all implicated in how crashes of complex vehicles occur.
Anyone who has ever been in a car crash probably agrees that correctly identifying exactly what happened is a challenging task, at least. How can the multiple, parallel conditions present in driving and car crashes be conceptualised? Rather than an ‘ethics of driver-less cars’ as a set of programmable rules for appropriate action, could it be imagined as a process by which an assemblage of people, social groups, cultural codes, institutions, regulatory standards, infrastructures, technical code, and engineering are framed in terms of their interaction? Mike Ananny suggests that “technology ethics emerges from a mix of institutionalized codes, professional cultures, technological capabilities, social practices, and individual decision making.” He shows that ethics is not a “test to be passed or a culture to be interrogated but a complex social and cultural achievement” (emphasis in original).
What the Trolley Problem scenario and the applications of machine learning in driving suggest is that we’re seeing a shift in how ethics is being constructed: from accounting for crashes after the fact, to pre-empting them (though, the automotive industry has been using computer simulated crash modeling for over twenty years); from ethics that is about values, or reasoning, to ethics as based on datasets of correct responses, and, crucially, of ethics as the outcome of software engineering. Specifically in the context of driverless cars, there is the shift from ethics as a framework of “values for living well and dying well”, as Gregoire Chamayou puts it, to a framework for “killing well”, or ‘necroethics’.
Perhaps the unthinkable scenario to confront is that ethics is not a machine-learned response, nor an end-point, but as a series of socio-technical, technical, human, and post-human relationships, ontologies, and exchanges. These challenging and intriguing scenarios are yet to be mapped.
Maya is a PhD candidate at Leuphana University and is Director of Applied Research at Tactical Tech. She can be reached via twitter
(Some ideas in this paper have been developed for a paper submitted for publication in a peer-reviewed journal, APRJA)
Source: Redditblog.com
Users and administrators alike constantly refer to Reddit as a community. Whether talking about specific subreddits or the site as a whole, the discourse of community is powerful. Unlike Facebook or Twitter, it isn’t just a branding concept. Many Reddit users also consider Reddit a community in a way other sites are not. Redditors appreciate that the site isn’t a social media network. They like that the model for Reddit is about content aggregation and forum discussion, they like the relative anonymity they have, and they like being able to curate their experience by subscribing to subreddits tailored to their interests.
I have previously argued that Facebook is not a community. I feel less confident making that argument for Reddit, primarily because so many users consider it a community. Regardless of my own definition of community—a social unit based on voluntary association, shared beliefs and values, and contribution without the expectation of direct compensation—and the extent to which it does or does not fit this definition, the fact is that there is an important affective component to community, and many users certainly feel that connection. more...
“We need to tell more diverse and realistic stories about AI,” Sara Watson writes, “if we want to understand how these technologies fit into our society today, and in the future.”
Watson’s point that popular narratives inform our understandings of and responses to AI feels timely and timeless. As the same handful of AI narratives circulate, repeating themselves like a befuddled Siri, their utopian and dystopian plots prejudice seemingly every discussion about AI. And like the Terminator itself, these paranoid, fatalistic stories now feel inevitable and unstoppable. As Watson warns, “If we continue to rely on these sci-fi extremes, we miss the realities of the current state of AI, and distract our attention from real and present concerns.” more...
Like raising kids, there is no handbook that tells you how to make the thousands of decisions and judgment calls that shape what a conference grows into. Seven year into organizing the Theorizing the Web conference, we’re still learning and adapting. In years past, we’ve responded to feedback from our community, making significant changes to our review process (e.g., diversifying our committee and creating a better system to keep the process blind) as well as adopting and enforcing an anti-harassment policy.
This year, we’ve been thinking a lot about what we can do to ensure that presentations respect the privacy of the populations they are analyzing and respect the context integrity of text, images, video, and other media that presenters include in their presentations. I want to offer my take—and, hopefully, spark a conversation—on this important notion of “context integrity” in presenting research.
In “Privacy as Contextual Integrity,” Helen Nissenbaum observes that each of the various roles and situations that comprise our lives has “a distinct set of norms, which governs its various aspects such as roles, expectations, actions, and practices” and that “appropriating information from one situation and inserting it in another can constitute a violation.” It’s often social scientists’ job to take some things out of context and bring understanding to a broader audience. But, how we do that matters. more...
Making the world a better place has always been central to Mark Zuckerberg’s message. From community building to a long record of insistent authenticity, the goal of fostering a “best self” through meaningful connection underlies various iterations and evolutions of the Facebook project. In this light, the company’s recent move to deploy artificial intelligence towards suicide prevention continues the thread of altruistic objectives.
Last week, Facebook announced an automated suicide prevention system to supplement its existing user-reporting model. While previously, users could alert Facebook when they were worried about a friend, the new system uses algorithms to identify worrisome content. When a person is flagged, Facebook contacts that person and connects them with mental health resources.
Far from artificial, the intelligence that Facebook algorithmically constructs is meticulously designed to pick up on cultural cues of sadness and concern (e.g., friends asking ‘are you okay?’). What Facebook’s done, is supplement personal intelligence with systematized intelligence, all based on a combination or personal biographies and cultural repositories. If it’s not immediately clear how you should feel about this new feature, that’s for good reason. Automated suicide prevention as an integral feature of the primordial social media platform brings up dense philosophical concerns at the nexus of mental health, privacy, and corporate responsibility. Although a blog post is hardly the place to solve such tightly packed issues, I do think we can unravel them through recent advances in affordances theory. But first, let’s lay out the tensions. more...
In his recent open letter to the “Facebook community,” Mark Zuckerberg issues a call to arms for people around the globe to come together in service of amorphous ideals like safety and civic engagement. He uses the term “community(ies)” over 100 times in the post.
He keeps using that word. I do not think it means what he thinks it means.
Community is one of those words that gets applied to so many social units that it becomes practically meaningless. Facebook is a community. The city you live in is a community. The local university is a community. Your workplace is a community. Regardless of the actual characteristics of these social units, they get framed as communities. But more often than not, they are not communities. This is not merely a semantic distinction; it has important consequences for how we think about governance, scales of human interaction, norms and values, and politics.